AI Winter: A Cycle of Over-Promising & Under-Delivering AI Technology
May 22, 2023 | 6 minutes read
Over-Promising, Under-Delivering
We’ve all been there before. You see an advertisement for a shirt that looks amazing on the model and has a slew of five-star ratings on the website, all of which makes paying an absorbent amount of money for the product worth it. Except when the shirt finally arrives, the fabric’s color is off, it doesn’t fit right, and there is no way to get your money back because of the store’s no-return policy. For a few months, maybe a few years, you stop shopping for shirts online and instead focus on buying from stores where you can go inside and try on the shirt, ensuring that what you want in a shirt is actually attainable and not an over-hyped advertisement online.
The same thing applies to AI or artificial intelligence. Creators of complex AI systems have over-promised what their programs can do in the past, encouraging high hopes in consumers, before ultimately letting consumers down. When consumers are disappointed, they stop spending their money on products that may work, opting for the tried-and-true products that will work. And when consumers stop spending their money, so do investors.
AI Winter is when consumer interest in artificial intelligence is low and therefore investors stop investing money in artificial intelligence development. It is a time when few advancements are made in AI technology and technological progress stands still. As consumers begin yearning for technology that can think for itself, making their daily lives and work easier, money from investors begins trickling into the industry once again, leading to new discoveries and advancements in technology – this time period is referred to as AI Summer.
The Imitation Game
Years before the term “artificial intelligence” would be coined by John McCarthy from MIT, there was Alan Turing. If his name sounds familiar, that’s probably because Turing was a cryptanalyst during World War II, who helped develop the Bombe machine which broke German ciphers created by the Enigma machine, aiding the Allied forces in winning the war. In 1950 Turing published a paper that focused on the question: can computers think like a human?
The Turing Test, as it became known, was a game of imitation. If a computer and a human were to both answer a question, could a judge who knew one response came from a computer accurately pick out which answer was man-made and which was machine-made? It has been 73 years since the Turing Test came into existence and no piece of artificial intelligence technology has managed to pass it but that doesn’t mean AI hasn’t come a long way since WWII.
The Golden Years of AI
The beginning of the golden years of AI was largely government-funded due to interest in MT or machine translation. On January 7th, 1954 the results of the Georgetown-IBM Experiment were unveiled in New York. The New York Times called it “[a] public demonstration of what is believed to be the first successful use of a machine to translate meaningful tests from one language to another. . .” At the height of the Cold War, the instantaneous translation of Soviet documents and communications in Russian was of utmost importance to the United States government, making this demonstration of MT one of true significance.
The Georgetown-IBM Experiment took place on a military-grade computer which was programmed with six grammatical rules and 250 vocabulary words that applied to the topics of organic chemistry, politics, law, and military affairs. The demonstration was a huge success, and one of the top linguists behind the Georgetown-IBM Experiment, Dr. Leon Dostert, predicted that within three to five years, MT could be advanced enough to produce meaningful translations in multiple languages on a variety of topics.
The US government, keen to beat the Soviet Union in everything from landing on the moon to producing the best nuclear weapons, invested heavily in machine translation. However, like everyone who has shopped online at some point, they were greatly disappointed with the outcome.
The First Winter & A Japanese Summer
The kickoff of the first AI Winter began with the publication of a report, known as the Automatic Language Processing Advisory Committee or ALPAC report. The ALPAC report concluded machine translation was not as cost-effective as using the slew of trained, human translators and therefore was of no use to the government or the general public at that time. After the ALPAC report was published, government investment in MT dwindled, and therefore progress in developing the necessary technology to improve machine translation slowed.
While US government spending and enthusiasm towards artificial intelligence waned, the Japanese government put more than a billion dollars towards research and development of the Fifth Generation Computer System or FGCS project throughout the 1980s. Instead of relying on artificial intelligence that was based on complicated algorithms, the FGCS project hoped to develop AI that was able to make inferences based on a large base of knowledge.
For instance, if the AI knows that a Doberman is a type of dog and that all dogs bark, it will know that Dobermans bark. This inference-based AI is known as KIPS or Knowledge Information Processing Systems and was an entirely new development in the field of computer technology.
While the Japanese FGCS project was successful in developing KIPS machines that were unlike any of the artificial intelligence technologies that existed at the time, it failed to produce something better than what there already was. It was yet again another case of over-promising and under-delivering on the capabilities of artificial intelligence.
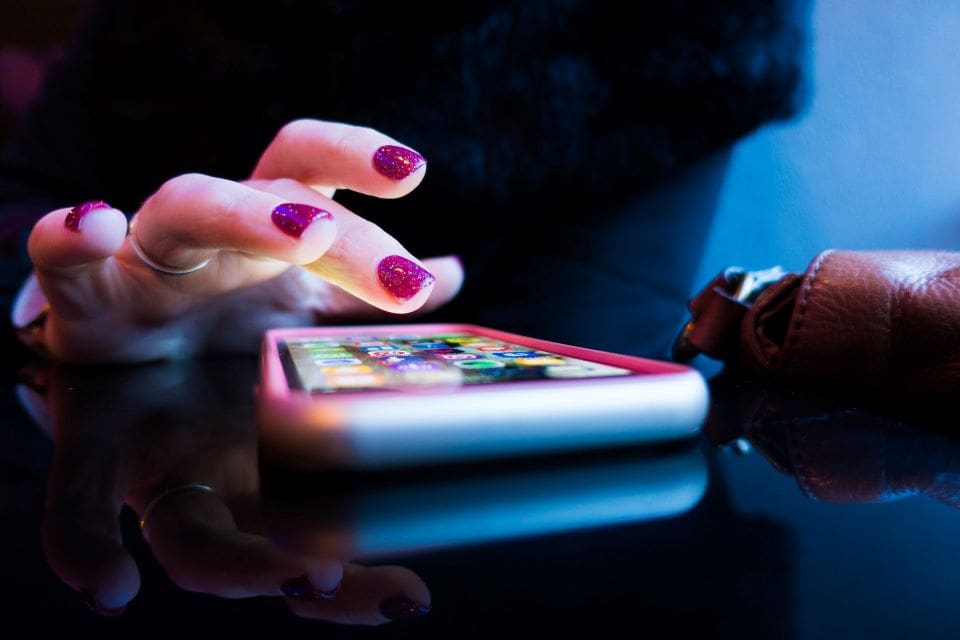
Twenty Years of Summer and Counting
The turn of the twenty-first century was a wild time for technology development. The internet had become increasingly popular, we saw the introduction of camera phones and Bluetooth-capable devices to the consumer marketplace. In 2023, nearly everyone has a smartphone with a camera and own a device with Bluetooth but in the late 90s and early 2000s, they were state-of-the-art technology that gave the general public a yearning for more. Thanks to the consumer interest in advanced technology, there was an influx of spending that enabled AI Summer to shine once again and it hasn’t stopped yet.
Nowadays, artificial intelligence is everywhere. There are AI assistants like Siri and Alexa, text-composing applications like ChatGPT, and self-driving cars and buses hitting the road. Machine translation has even made a comeback from its earlier exile with programs like Google Translate and Microsoft Translator.
AI Redaction
It’s no surprise that over seventy years since Alan Turing first posed the question: can computers think?, that AI has become a staple in helping complete tasks that otherwise would require a great deal of time and attention from humans. One of these tasks is redaction. Redacting video, audio, image, and document files by hand can be a tedious task but with the help of AI Automatic Detection, Automatic Transcription, and Automatic Translation, redaction doesn’t have to be difficult.
CaseGuard Studio is an all-in-one redaction software that uses artificial intelligence and machine translation to help law enforcement agencies, hospitals, schools, and many other industries accurately and efficiently redact sensitive information from their files. Even if the current AI Summer turns into an AI Winter, CaseGuard Studio’s AI capabilities will continue to advance in order to provide the best redaction software possible.