How Can Machine Learning be Used to Fix Unconscious Bias?
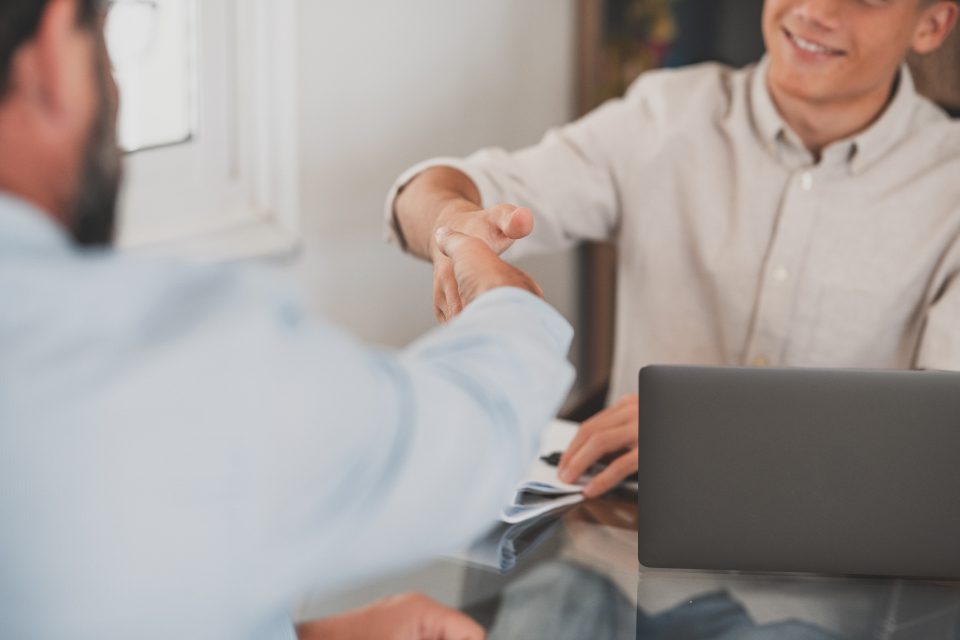
As machine learning models and AI systems have come to influence many aspects of American society, from the criminal justice system to immigration decisions, it is imperative that these methods are free from bias. As almost all people hold some form of bias at one point or another, this can prove to be exceedingly difficult. What’s more, many of the teams who develop the data models used in machine learning algorithms are not themselves diverse. It is impossible to represent every demographic in a data set if the set has been created by a single demographic. In such a scenario, issues will invariably occur given enough time.
While there are a great number of challenges in the ways in which we detect unconscious bias in machine learning, there are some ways that machine learning can be used to positively impact unconscious bias. For example, prejudice bias is very common in American society. In the context of machine learning, a computer vision program may be implemented in the workplace that contains many women cooking and many men coding. Over time, this program would learn that men are coders and women are chefs, despite the fact that men can be chefs and women can be coders.
How can Machine Learning be used to fix humans’ unconscious bias?
One area where unconscious bias is most present is in hiring decisions made by businesses, corporations, or other employers. For instance, an employer may have an unconscious bias towards a certain race or ethnicity of people and subconsciously avoid hiring people from these groups. Alternatively, as some occupations such as athletics and manual labor positions have long been associated with masculinity, someone hiring for these jobs may not consider a woman at all. Finally, age bias can also be prevalent in hiring decisions, as an HR professional making a hiring decision may feel like a younger person fresh out of college is easier to work with when compared to an older person who may have an established work style.
Let’s take the resumes as an example, machine learning algorithms can be used to censor resumes’ personal and identifiable information altogether. It can detect and redact names, addresses, phone numbers, email addresses, schools names, and dates so that hiring managers can make hires based on skills, education, and ability.
Moreover, machine learning capabilities can be used to both expedite and improve upon this process, as the algorithms will learn over time. Automatic redaction technology makes this process both intuitive and seamless, as manually redacting all of this information from resumes would take an unreasonable amount of time. Additionally, a hiring manager may want to take this redaction further by censoring an applicant’s GPA or the school they attended, an attempt to ensure that a decision is made entirely on merit without any other extenuating factors.