Facial Recognition Technology And Your Privacy
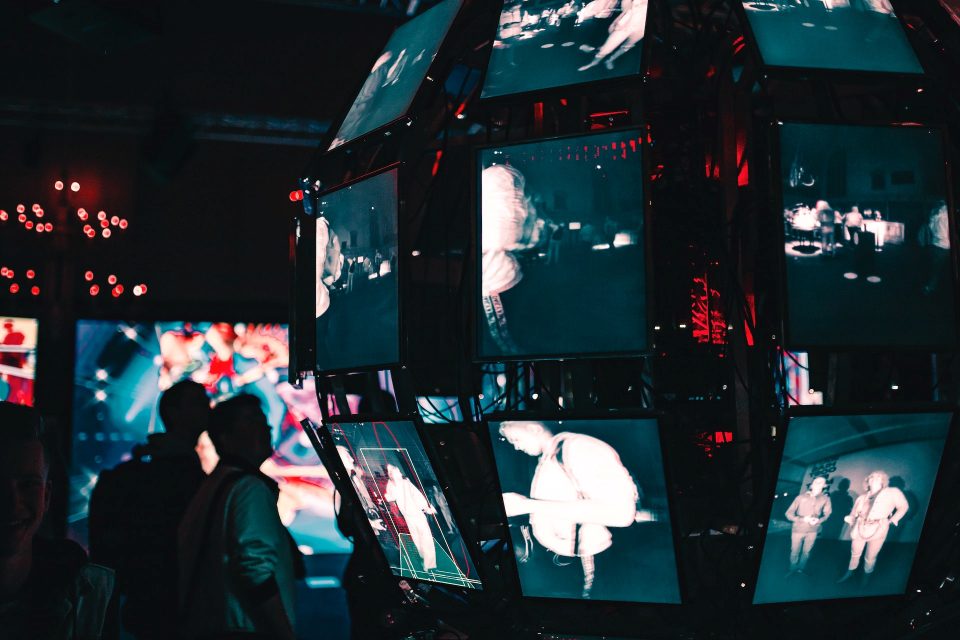
We have all witnessed the tense scene in spy movies where investigators exhaustively sift through a live surveillance feed in the hopes of finding the villain’s face amongst thousands of other faces in the crowd. The scene intensifies when a voice behind the computer yells “Target identified” followed by sharing their monitor on the big screen for the rest of the room to observe. On the live feed, a box appears around the person’s face as they move through the crowd alongside a static picture of the person, on the side of the screen, with the caption that reads “100% match.”
The agents on the ground rush into the crowd, chase the villain, and eventually capture them. It’s a job well done, but how did they identify the target in a crowd of a thousand people? The answer lies in facial recognition technology.
Facial recognition technology is not just in the movies, it’s in our phones, used at customs checkpoints, and is a hot-button topic when it comes to protecting personal privacy.
What is Facial Recognition Technology?
Facial recognition technology (FRT) is programmed to determine the similarity between two facial images as a way of identifying a match. The software accomplishes this by identifying and quantifying the facial features so that they can be used for comparison when the program is presented with a second image of a face.
FRT uses machine learning to identify and discern between objects like faces and license plates from one another. The software turns unique facial features like eyes, noses, and mouths into numeric data points for cross-comparison. The algorithm within FRT produces a similarity score when data points from one face are compared to another set of data points from a different face. This similarity score informs the humans overseeing the confidence level of the FRT in matching or differentiating between two faces. If an object like a license plate is in the same image or video as a face, FRT will recognize the license plate’s distinct lack of recognizable facial features and excludes it from any facial analysis.
Accuracy & Algorithmic Bias
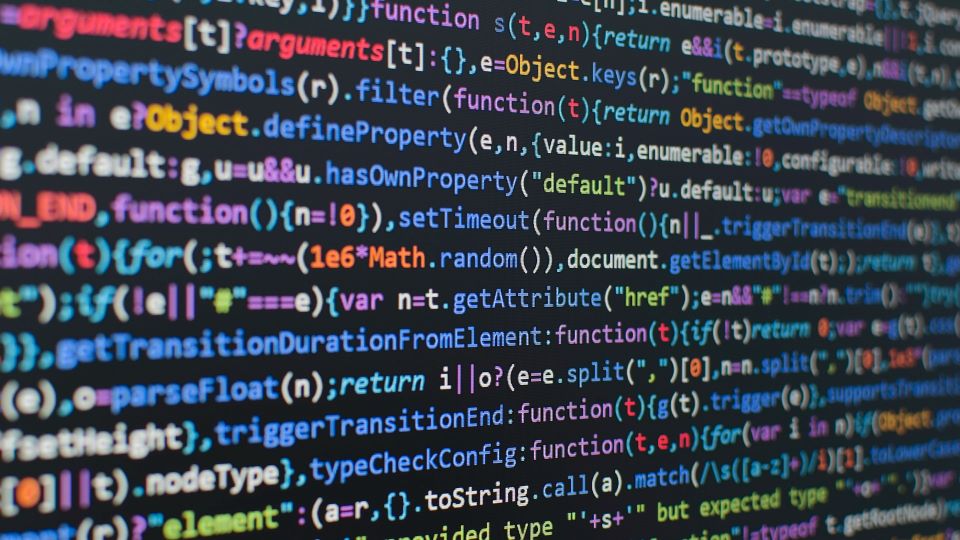
The accuracy of facial recognition technology depends on the circumstances in which it is used. When comparing images of two faces in similar lighting situations and angles, FRT software has a high degree of accuracy. However, if one image is a mug shot, for example, taken under fluorescent lighting with a high-quality resolution compared to a grainy still captured from black and white security camera footage, the degree of accuracy will fall due to the less-than-ideal circumstances.
Furthermore, the accuracy of FRT software also depends on the images that it was trained on during the initial machine learning process. If the majority of images depicting faces from only one demographic, then the program would be more adept at accurately identifying the features of that particular demographic and less accurate when prompted to identify and compare the features of a face from a different demographic, leading to a strong racial bias.
According to a December 2019 study from the National Institute of Standards and Technology (NIST), researchers found that among the 100+ FRT software tested, program accuracy was highest for white, middle-aged, men. The differences between accurately identifying a white male versus a black female were significant; the facial recognition technology software tested falsely identified images of black females anywhere between 10 and 100 times more frequently than it falsely identified images of white males.
An explanation for algorithmic racial bias could be a result of a non-diverse data set used during the machine learning process. This issue, however, can be addressed and rectified. Ongoing research by NIST and the Department of Homeland Security shows a significant increase in accuracy across all demographics as of 2023. Despite these improvements, concerns about misidentification continue due to the real-world legal implications that can result from such errors.
Nijeer Parks, a 33-year-old African American man was arrested in February 2019 and accused of shoplifting candy and trying to hit a police officer with a car. Except, he was 30 miles away at the time of the incident. Mr. Parks was arrested due to a false match by facial recognition technology used by state investigators in New Jersey. According to Mr. Parks, the only similarity he saw between the photo, used to wrongly accuse him of the crime and himself, was that they were both African American men with beards. After spending 10 days in jail and $5,000 on legal fees, the case was dismissed for lack of evidence.
With the known racial bias of FRT algorithms and the rising tensions between law enforcement and minority communities, it is not surprising that groups such as the ALCU believe that FRT could be used to target Black and Brown communities in the United States. A month after the death of George Floyd, IBM, Amazon, and Microsoft all announced that they would pause or stop the sale of their facial recognition technology to law enforcement agencies in part because of its potential use for racial profiling. Despite increased accuracy since the 2019 NIST study, legal action has been taken to restrict FRT usage by law enforcement and government agencies.
Privacy Concerns
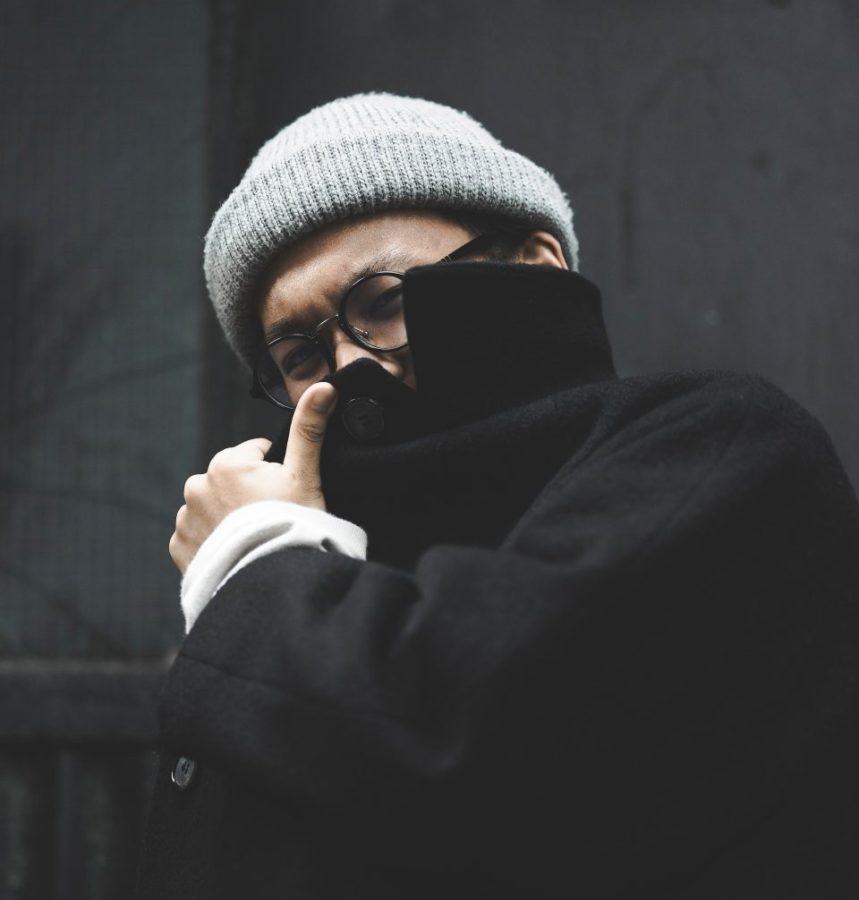
Several U.S. cities have put bans or limitations on the use of facial recognition technology by law enforcement and government agencies due to the aforementioned potential for racial profiling and misidentification of subjects. Congress has yet to pass legislation regulating the use of FRT, leaving it to state and city governments to handle. However, this does not mean that Congress has not been trying. Senators Coons and Lee of the 116th Congress introduced the Facial Recognition Technology Warrant Act of 2019, which would have required law enforcement agencies to obtain a warrant before using FRT, but the bill died in committee without a vote. Due to the lack of federal regulation, nearly two dozen state or local governments between 2019 and 2021 put legislation restricting the use of FRT on the books but with concern over rising crime rates, some states have begun to roll back their anti-FRT legislation to help investigators.
In part due to the controversial domestic implementation of facial recognition technology in the U.S., China has taken the lead as the world’s exporter of FRT. The possible political implications of China’s exportation of facial recognition software have been noted by academics at Harvard and MIT in a combined report, where it is argued that increased government surveillance could turn weak democracies into full-blown autocracies and could be a helping hand in increasing human rights abuses within those countries.
The Bright Side of FRT
With all of the concerns over privacy violations regarding facial recognition technology, we cannot disregard how FRT can be used to protect personal privacy. CaseGuard Studio, an all-in-one redaction solution uses an AI-powered facial detection feature to allow its users the ability to automatically detect and redact faces from images, videos, and documents.
Redaction serves as an important tool for providing anonymity, whether it’s passersby caught in surveillance footage of a crime scene, students uninvolved students in a classroom altercation, or million other scenarios requiring faces/identities to be concealed. Without AI-powered FRT, members in police departments, schools, hospitals, and many other agencies would have to painstakingly manually redact faces from images, videos, and documents before publicly releasing them. While manual redaction gives those performing redactions total control, it is undeniably time-consuming. In contrast, CaseGuard’s AI Automatic Detection ensures the protection of the privacy of individuals in a very quick and efficient manner.