What is Tiny ML? What are its Business Applications?
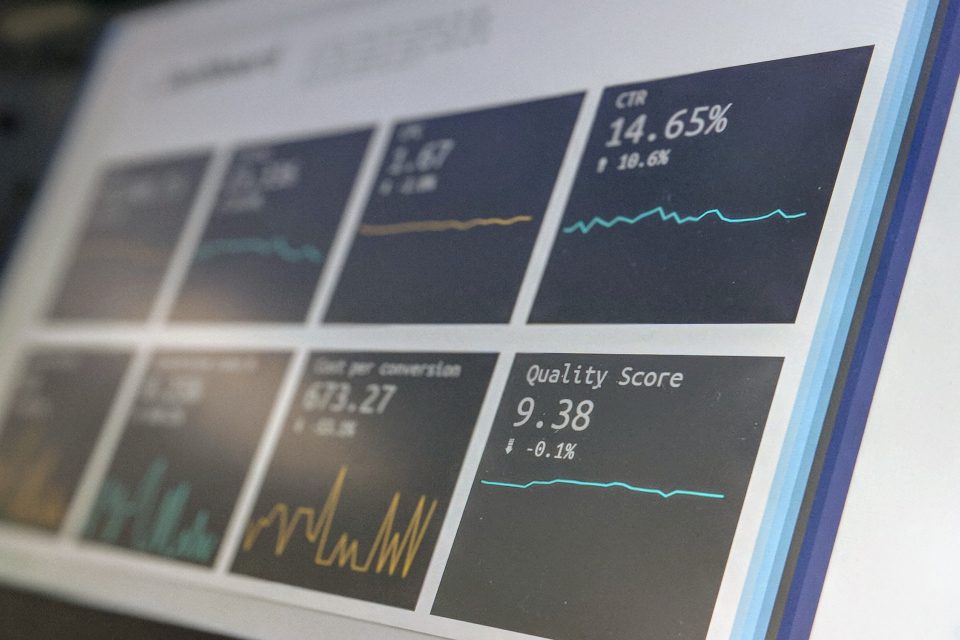
Despite the incredible advancements that have been made through the development and use of artificial intelligence and machine learning algorithms, the processes that are necessary to adequately create these applications can be extremely costly and time-consuming in practice.
To illustrate this point further, major multinational technology companies such as Google and Microsoft have spearheaded the creation of products and services that utilize AI and machine learning models in recent years, including Google’s Bidirectional Encoder Representations from Transformers (BERT), among others, as these companies have the resources and raw data needed to develop such applications.
For these reasons, many software developers and engineers have looked to Tiny ML when trying to create new applications and services. Tiny ML refers to “a field of study in Machine Learning and Embedded Systems that explores the types of models you can run on small, low-powered devices like microcontrollers.”
As a result, the small nature of Tiny ML models means that they can function using far less computational power, latency, and bandwidth than typical machine learning algorithms. Furthermore, these models can also be run using cloud and edge computing, meaning that the personal data that are used to train such models won’t need to be stored in a server.
The benefits of Tiny ML
To this last point, the Tiny ML approach provides many benefits to software developers as it concerns the costs and speeds that are typically associated with creating a machine learning model. For example, as has already been stated, the fact that Tiny ML models can be run using less computational resources than more traditional approaches to machine learning means that these models can run for weeks if not months at a time by simply using batteries.
Likewise, smart AI assistants such as Amazon’s Alexa and Google Now are perhaps some of the most common examples of Tiny ML within our current business landscape.
This being said, a smart assistant such as Alexa does not need a CPU or GPU to function effectively, as consumers that purchase such devices can simply charge the smart assistant just as they would for any other household appliance.
What’s more, the low level of latency that is part and parcel of Tiny ML models enables consumers to receive a swift and expedient response when talking to a smart assistant such as Alexa, in contrast, to many other software applications that function in accordance with inference server that must send and receive data in order for the system to function properly.
On top of this, Tiny ML models are far less expensive to produce when compared to artificial neural networks, supervised learning algorithms, reinforcement learning models, etc. For reference, training a large language model such as Google’s BERT or Open AI’s Generative Pre-trained Transformer (GPT) 3 can cost hundreds of thousands of dollars, even before considering the data and devices that are needed to facilitate such training.
Due to this fact, the overwhelming majority of software developers will not be able to afford to train similar models, as they will not be able to access the same level of resources that are afforded to developers that are employed by major technology companies.
Applications of Tiny ML in the business world
With all this being said, while AI assistants such as Amazon’s Alexa and Google Now are some of the most common applications of Tiny ML within the business world today, there are countless other ways in which this approach can be implemented within a wide range of other business models.
For instance, Tiny ML is currently being used within the field of healthcare to help reduce the spread of mosquito-borne diseases such as Malaria, Zika Virus, and Dengue, in addition to others. To accomplish this, devices are placed in specific water sources and trained on data that has been observed regarding mosquito breeding conditions. Subsequently, these devices are powered using solar energy and as a result, can be run indefinitely.
On the other hand, another way in which Tiny ML is currently being used within the business landscape is in the world of agriculture. For example, the Nuru app, described as “a publically supported, and publically developed application that uses a digital assistant to help farmers diagnose crop disease in the field, without an internet connection”, is currently being used by farmers around the globe in order to combat crop failure.
To this end, the app “uses Google’s Tensorflow machine learning tool and a database of images collected by crop disease experts across the world.” Moreover, due to the fact that the application does not need an internet connection in order to function, it can be used in various different climates and weather conditions without issue.
While smart AI assistants, healthcare, and agriculture are just a few sectors within the business world in which Tiny ML is currently being used to aid in the development of new products and services, there are countless other applications of this groundbreaking technology in other fields of business as well.
This is due in large part to the ways in which Tiny ML has made artificial intelligence more accessible to the general public, as the approach lends itself to software engineers that may be working with limited resources, relatively speaking. For this reason, Tiny ML will likely continue to be used throughout the business world for years to come.