The New York Times, Machine Learning, and Paywalls
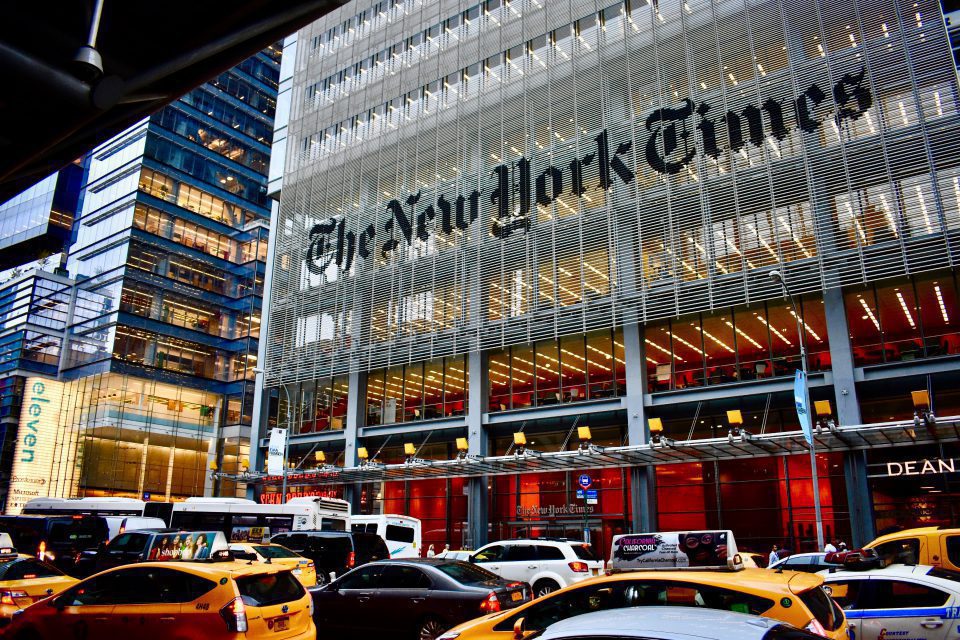
Have you ever been hit with an unexpected paywall when reading an online article about a particular current event or topic of interest? Despite the fact that journalists and editors write and produce articles and other forms of content that consumers read on a daily basis, the news companies that employ these creatives must ensure that they are able to monetize their online platforms in the most effective and efficient way possible. For this reason, the New York Times, one of the most established and renowned daily newspapers in the world, has been using machine learning algorithms to identify which of their readers will be most likely to subscribe to their website when browsing free online articles.
More specifically, the NYT uses a machine learning algorithm referred to as the Dynamic Meter, which essentially works to make the paywall of the newspaper more intelligent and intuitive. To this point, a blog post written by Rohit Supekar, a data scientist that works for the NYT’s algorithmic targeting team, explained that the Dynamic Meter has been configured to show online readers “a registration wall that blocks access and asks them to create an account. This allows them access to more free content, and a registration ID allows the company to better understand their activity. Once registered users reach another meter limit, they are served a paywall with a subscription offer.”
Digital subscriptions
In spite of the fact that digital subscriptions have become the norm in recent years as more and more people look to online sources for news and general information, this change to the ways in which content is currently consumed did not occur overnight. Subsequently, even a newspaper such as the New York Times, which boasts 7.8 million subscribers across both its digital and print platforms, did not exert major focus on the online landscape until a little over a decade ago. Likewise, in 2011, the NYT made a concerted effort to put more emphasis on its digital features, in accordance with metered access that was intended to provide non-subscribers with a set number of opportunities to engage with the newspaper’s content before registering and ultimately, signing up for a paid subscription.
Through the concept referred to as the subscription funnel, the NYT was able to continue growing its subscriber base, even as many other major newspapers around the world struggled to contend with new content creators that were emerging within the digital space. For these reasons, the predictive nature of machine learning made this subfield of artificial intelligence a perfect fit for the New York Times, as having a dedicated team of employees work to determine which online readers would be most likely to pay for an NYT subscription would be an arduous and painstaking task. Alternatively, the sheer amount of data that the NYT has gathered about its readership during the course of its 100-plus-year history makes training a machine learning model extremely easy.
Casual artificial intelligence
What’s more, in addition to predicting which online users will be most likely to sign up for a New York Times subscription, the Dynamic Meter machine learning model also works to understand what would have happened if the algorithm had chosen another portion of the newspapers readership base to extend additional free articles to. To illustrate this point further, while many major businesses and corporations offer free or very inexpensive items to their customers as an incentive for them to purchase more goods and services, such as the McDonald’s Dollar Menu, among other things, it can be very difficult to establish what threshold of free or reduced-price items will provide consumers with the impetus to spend more of their money with a given business or organization.
In the context of the New York Times, the paper’s writers and editors were having trouble deciding which number of articles they should allow non-subscribers to read for free before urging them to sign up for a subscription. To solve this problem, the Dynamic Meter conducted a series of randomized controlled trials, where certain groups of readers were given an arbitrary number of free articles before being prompted to either create an account or begin paying for a subscription. Through these randomized trials, the New York Times was able to observe the engagement rate of a certain percentage of its user base, and then train the Dynamic Meter in conjunction with these new findings and insights. This being the case, the algorithm was able to account for the delicate balance between converting unpaid subscriptions into paid subscriptions while simultaneously offering free articles to online users who choose to stop engaging with the newspaper altogether.
As artificial intelligence and machine learning have completely changed the fashion in which many businesses and organizations generate revenue and profits, it is understandable that the world of online newspapers would be no different. Furthermore, due to the fact that newspapers are inherently full of data and information, they are the ideal medium for training a machine learning algorithm to identify a particular objective or task. As such, online users can rest assured that the paywalls they hit when reading a New York Times article are not at all random, even if such paywalls make it difficult for said users to read free content in their leisure time.