5 Applications of ANNs in the Business World
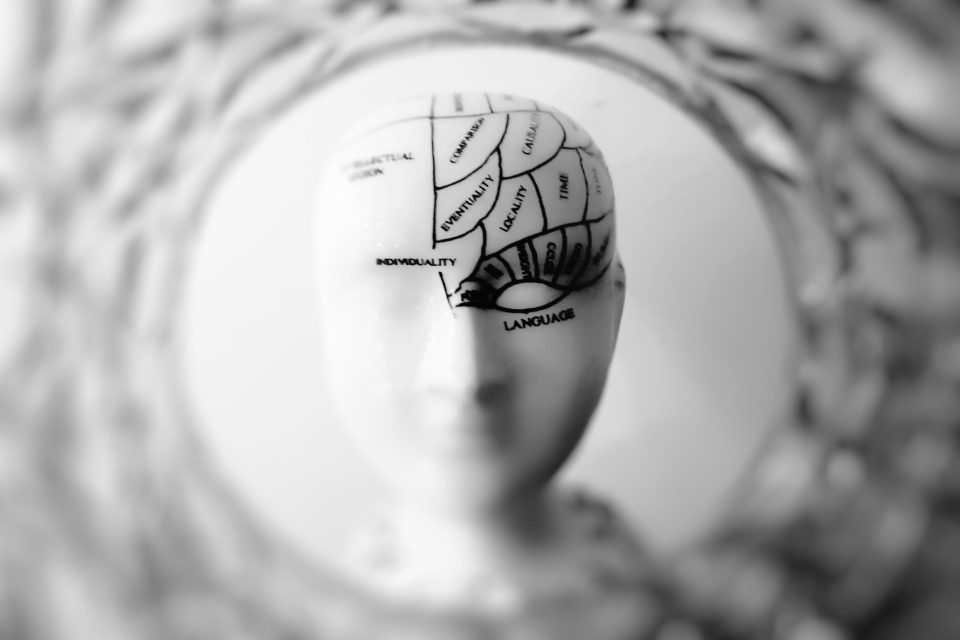
In the modern age of technology, the implementation of artificial intelligence and machine learning have enabled businesses to provide consumers across the globe with cutting-edge products and services that were thought to be impossible only a few decades ago. From major industries such as the American financial sector to more niche businesses that sell obscure retail products, the applications of AI and machine learning algorithms in the business world today are truly widespread and expansive. To this point, the structure and functionality of Artificial Neural Networks (ANNs) have given rise to a number of groundbreaking products and services. Likewise, some common applications of ANNs that are currently being used by business professionals include facial recognition software, stock market prediction, weather forecasting, healthcare, and signature verification/handwriting recognition.
Facial recognition software
Many facial recognition software programs that hinge on the ability of an application to recognize the occurrence of a human being’s face within a video, image, or photograph, such as automatic redaction software programs, among others, operate in conjunction with ANNs. More specifically, these kinds of software programs utilize Convolutional Neural Networks (CNNs), a class of ANNs that are geared toward analyzing visual imagery. This being said, the layers that are used to create CNNs are inspired by the organization of the human brain’s visual cortex, the primary region of the brain that enables human beings to receive and process various forms of imagery via the human visual system. Through this organization, CNNs can be configured to recognize the occurrence of a human face within a visual medium.
Stock market prediction
On the other hand, Multilayer Perceptrons (MLPs) have proven to be extremely effective at helping stock brokers make various predictions and decisions in relation to the stock market. MLPs are a class of feedforward neural networks, a very simple form of ANNs where the information passed through the numerous layers of said neural networks will be fed into one direction. To this end, an MLP that has been created to assist in the prediction of the stock market will contain multiple layers of nodes that correspond to certain financial metrics, including a stock’s past performances, non-profit ratios, and the annual returns of a particular stock or interest, among other pertinent information.
Weather forecasting
As weather forecasting has historically been a difficult undertaking due to the inherent unpredictability of the world’s multitude of regions and climates, ANNs can be used to aid meteorologists in forecasting future weather patterns in a few different ways. To illustrate this point further, CNNs can be combined with MLPs to aid in weather prediction, as the visual component of such predictions is directly applicable to CNNs, while the variety of factors that can influence the weather on a particular day, such as air temperature, humidity, solar radiation, and wind speeds, can be conveyed in an efficient manner through the use of MLPs.
Healthcare
Just as other types of machine learning and artificial intelligence algorithms have been developed for use in the healthcare industry, ANNs can also be used to help doctors tackle the wide range of issues they may encounter over the course of an average work day. Going back to Convolutional Neural Networks, these models can be very helpful in ultrasounds, CT scanning, and x-ray detection, just to name a few, due to the visual nature of these healthcare services. Moreover, many researchers that are employed by pharmaceutical companies have looked to Generative Neural Networks to assist them in discovering new drugs, as the costs associated with such tasks can be particularly prohibitive.
Signature verification/handwriting recognition
Lastly, another major application of ANNs in the business world is in signature verification and handwriting recognition. As digital signatures have become far more commonplace within the past 20 years, antiquated methods of handwriting recognition have instead been replaced with software programs that can do the same job virtually. To this end, the layers within an artificial neural network can also be trained to differentiate between authentic handwritten signatures and forged signatures, for both online and offline use. In such instances, a software developer will once again look to use a convolutional neural network to identify the various visual characteristics of a particular handwritten document or signature.
Despite the fact that facial recognition software, stock market prediction, weather forecasting, healthcare, and signature verification/handwriting recognition are just a few examples of implementations of artificial neural networks within the current business landscape, this landmark technology is also being used in many other business applications as well. Furthermore, as the computational power and resources that are needed to create artificial neural networks restrict their usage in certain industries from an economic standpoint, there are still many other ways in which this technology can be used for future business applications.